Implementation of a Clinical Decision Support System (CDSS)
in Psychiatry Based on Machine Learning (ML) and Reliable Datasets
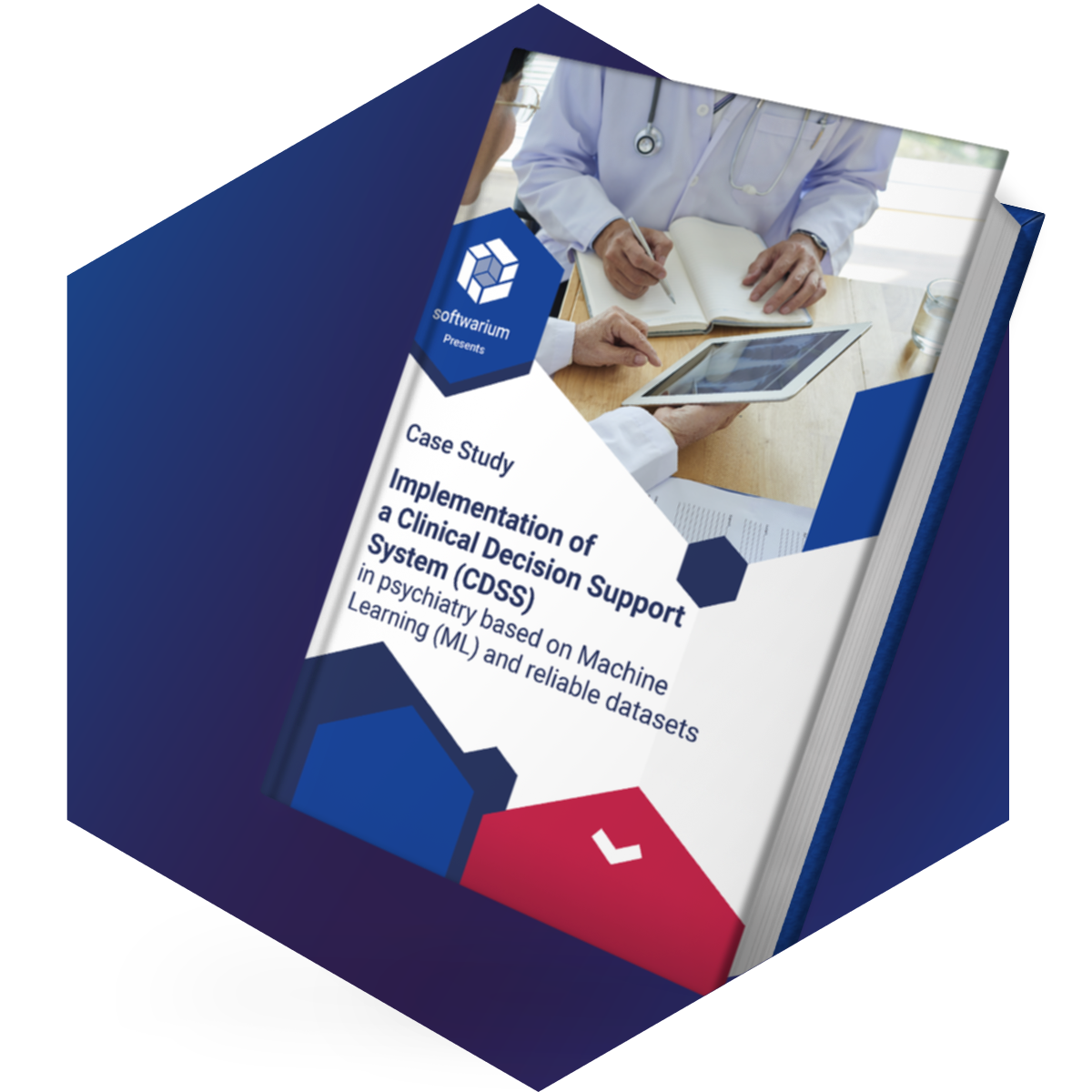
Solution Sneak Peek
Precision and Patient Safety
Identifying risk factors, mitigating medication errors, and optimizing drug-disease interactions to ultimately safeguard patients and to amplify their safety.
Evidence-Based Clinical Decisions
Our CDSS leverages ML to analyze patient data, medical history, and clinical guidelines.
Streamlined Clinical Workflows
The solution seamlessly integrates with electronic health record systems, so the clinicians gain instant access to patient-specific data.
Transparency and Trust
A system that not only delivers accurate recommendations but also offers clear explanations for its decisions. We empower clinicians to confidently embrace AI-driven insights.
Introduction
This case study focuses on the implementation of a clinical decision support system (CDSS) in psychiatry, showcasing the transformative impact of Machine Learning (ML) and reliable datasets.
A seamless integration of clinical decision support tools with electronic health records by Softwarium assisted healthcare providers in reducing medical errors, optimizing patient outcomes, and enhancing clinical decision-making based on evidence-driven guidelines.
Client's Vision
Our client recognized the critical role of clinical decision support systems in driving better patient safety and required a robust system that would leverage machine learning algorithms to provide relevant references to clinicians. The healthcare organization envisioned a CDSS that seamlessly integrates with existing clinical workflows, extracting and analyzing patient's medical history from digital health records to support diagnostic decision-making.
Motivated by improving patient safety and minimizing medication errors, the client asked Softwarium team to enhance their clinical management by empowering clinicians with accurate and up-to-date clinical guidelines.
We agreed that the impact of artificial intelligence and knowledge management in practical psychiatry is about to become transformative. CDSS can enhance diagnostic accuracy and treatment planning in psychiatric care. With the ability to analyze data, symptoms, and medical history of a patient, CDSS can provide valuable insights, identify patterns, and generate evidence-based recommendations. This can assist clinicians in making more informed decisions and tailoring treatment approaches to individual patients.
Understanding CDSS and ML
Clinical decision support system is a specialized software system designed to assist healthcare providers in clinical decision-making processes by providing context-specific knowledge and patient-related data reports.
Machine learning systems employ algorithms that learn from patterns and associations within the data, allowing the CDSS to make predictions and provide recommendations based on evidence-based medicine and clinical regulations.
By utilizing machine learning and advanced health information technologies, CDSS can analyze vast amounts of clinical data, including patient history, genetic testing data, and diagnostic data, to generate actionable insights.
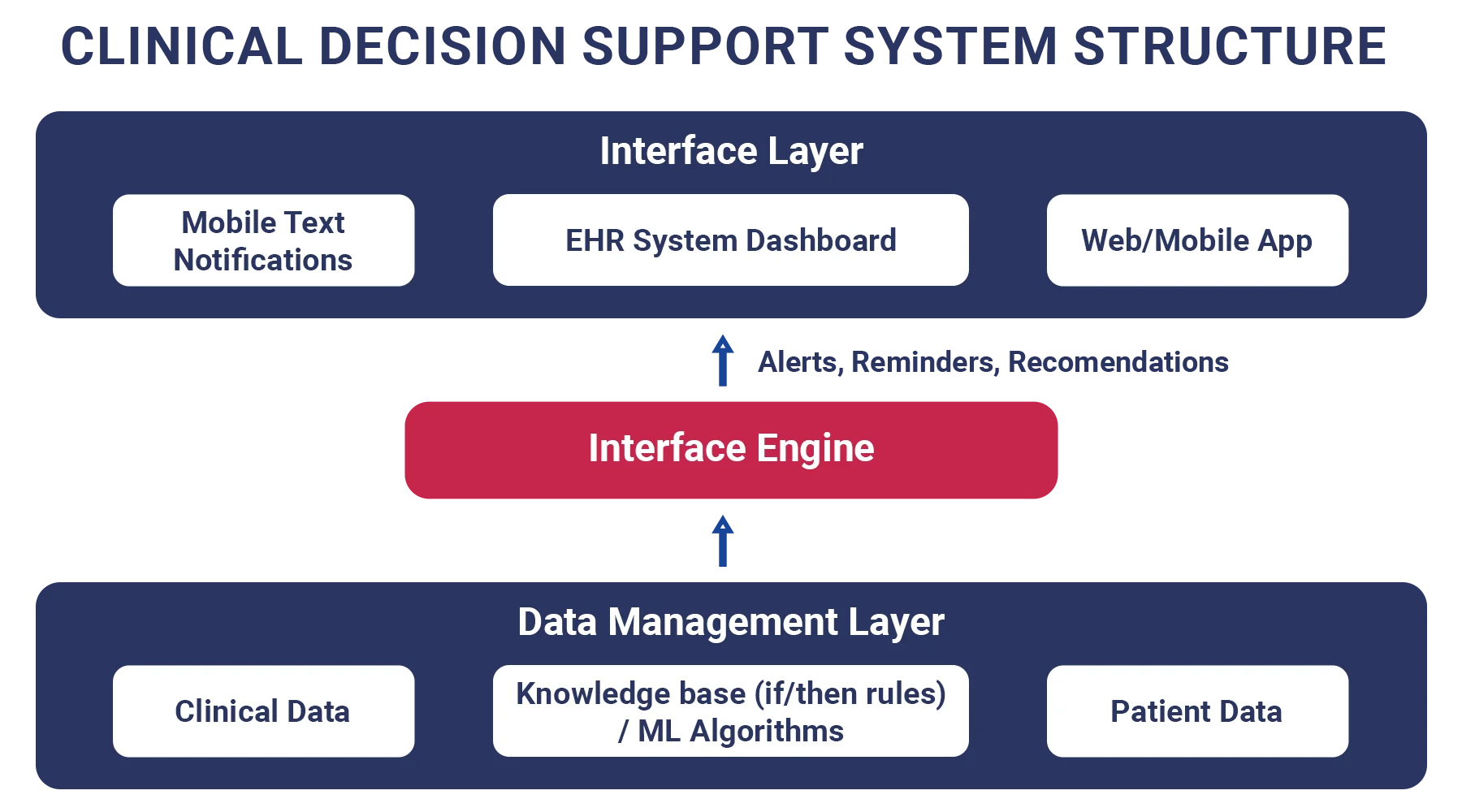
The scope of CDSS functions is extensive, covering areas such as drug prescription, diagnostics, disease treatment, notification systems, and drug dispensing. These functions contribute to patient safety, cost reduction, efficient clinical management, and streamlined administrative activities.
Building a CDSS involves considering various aspects. Patient data and medical knowledge are stored in an inference engine, which provides case-specific information and suggestions as needed. CDSS can be developed as a desktop or web application and can even be designed as a mobile app. Integration with existing IT infrastructure has to be seamless, ensuring compatibility and smooth implementation.
The Importance of Subject Competence
Developing an effective clinical decision support software requires subject-matter expertise in healthcare and machine learning, ensuring accurate data management and reliable decision support. This competence is crucial to developing CDSS software that aligns with clinical practice guidelines, minimizes alert fatigue, and provides clinically relevant recommendations to healthcare professionals.
Medical organizations that choose to collaborate with ML experts curate comprehensive and high-quality datasets that capture relevant patient data.
The Challenge
Our client faced challenges rooted in the limitations of existing systems and the potential for error in manual clinical decision-making. Without a functional CDSS, healthcare providers lacked efficient access to patient-specific medical knowledge and references. This hindered clinical workflows and compromised safety in healthcare.
The challenge was to overcome these limitations and implement a CDSS that would enhance health outcomes while mitigating risks associated with diagnostic inaccuracies.
The "Black Box" Concerns
In many cases, deep learning algorithms function as "black boxes," lacking the ability to provide explanations for the reasoning behind their conclusions. It becomes particularly significant in healthcare, where the well-being and lives of individuals are at stake. Without this transparency, it becomes challenging for healthcare professionals to place their trust in the recommendations provided by IT systems.
This raises critical questions: Can a computer truly replace human intuition? And who should be held accountable for the outcomes?
That's why our CDSS solution focuses on transparency and trust. We prioritize development of a system that not only provides accurate recommendations but also offers clear explanations for its decisions. By ensuring transparency and interpretability, we aim to build trust with clinicians and patients, empowering them to make clinical decisions confidently.
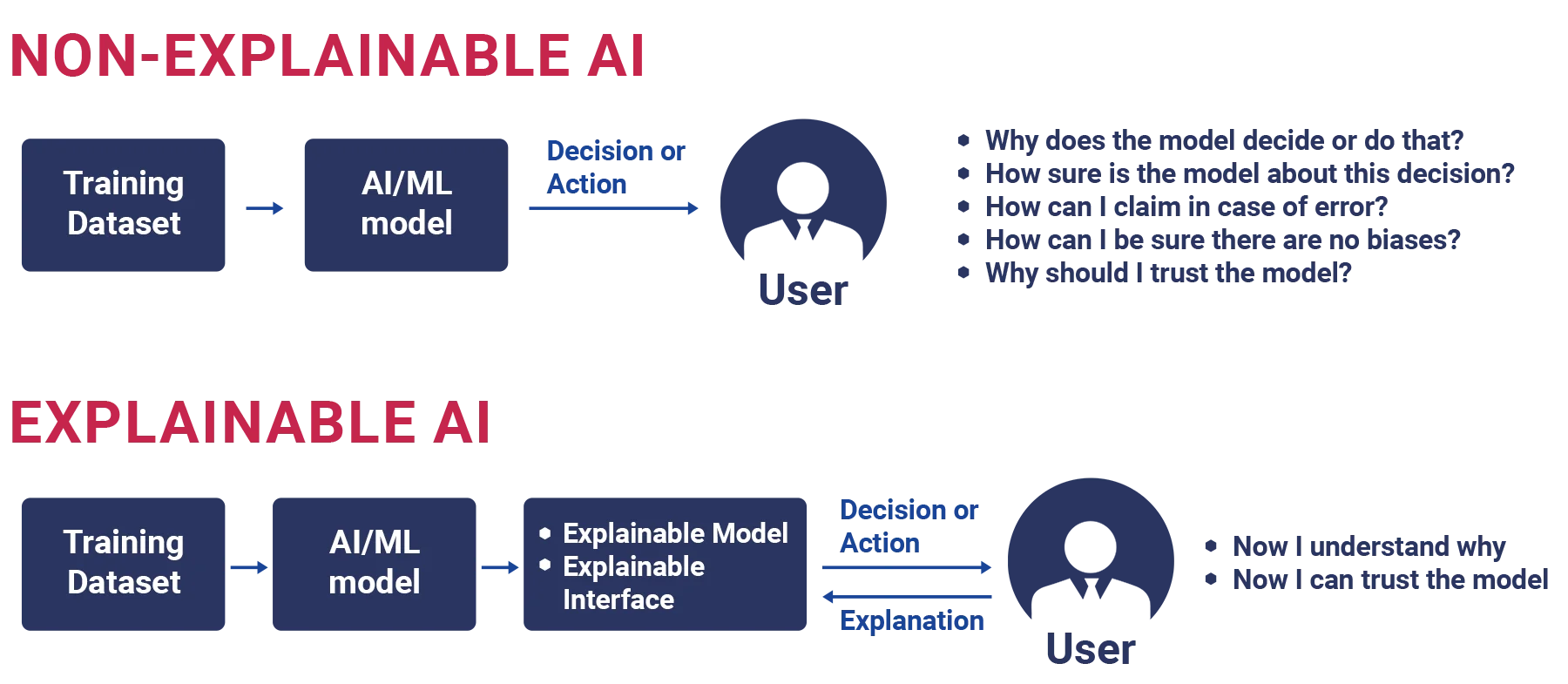
Objectives
The objectives of implementing the CDSS were multifaceted and aimed at revolutionizing the landscape of psychiatric care.
The primary objective was to boost patient safety by reducing medication errors through evidence-based decision support. By leveraging ML algorithms and clinical knowledge, the CDSS would provide healthcare professionals with contextually relevant recommendations, minimizing the risk of adverse drug events and ensuring optimal medication management.
Additionally, the CDSS aimed to enhance diagnostic accuracy by analyzing patient-specific data and generating evidence-based insights. By incorporating clinical guidelines and ML algorithms, the system would empower healthcare professionals with accurate and timely diagnostic support, leading to more precise and effective treatment plans.
Another key objective was to optimize clinical workflows by seamlessly integrating the CDSS with electronic health record systems. This integration would streamline access to patient-related data, allowing to make informed decisions efficiently. The CDSS would enable the generation of focused patient information reports, providing clinicians with comprehensive and contextually relevant information at their fingertips.
Ultimately, the overarching objective was to improve patient outcomes by leveraging advanced decision support tools, enhancing clinical decision-making, and ensuring personalized and evidence-based care pathways in the field of psychiatry.
The Solution
By leveraging machine learning systems and deep learning tools, we developed a CDSS that analyzed patient file from electronic health records, clinical documentation, and existing systems. The CDSS extracted and processed patient-specific data, identifying risk factors to provide diagnostic decision support and generate contextually relevant recommendations.
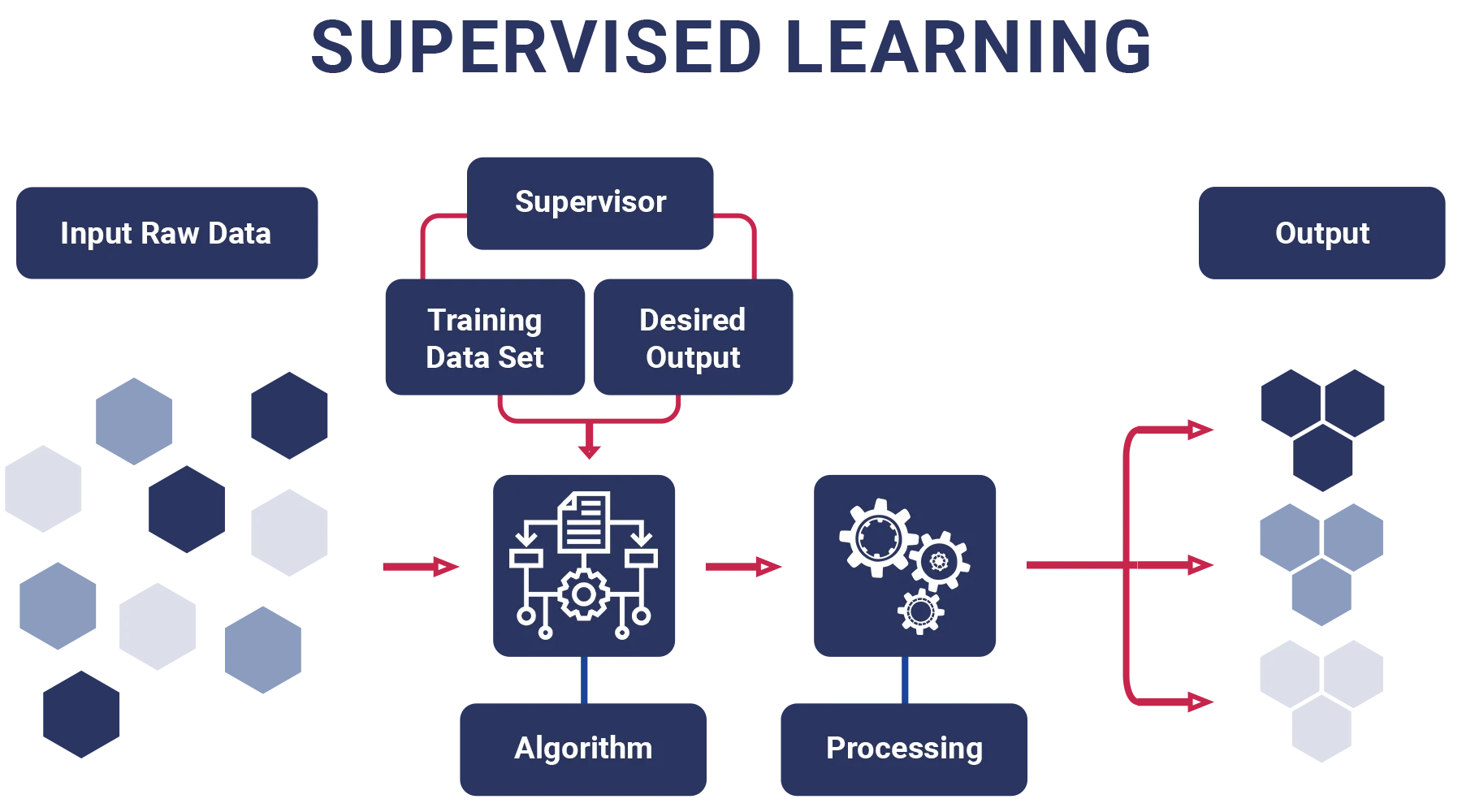
Softwarium team has successfully developed reliable CDSS datasets, referred to as "Golden Proof". By collaborating closely with subject-matter experts and utilizing Python tools, we converted raw data into structured inputs that align with clinical guidelines. These datasets encompass a comprehensive range of symptoms and diagnoses.
Implementation Process
The implementation process involved several key parts:
- Data Integration: Softwarium integrated the CDSS with electronic health record systems, enabling seamless extraction and analysis of focused patient data reports.
- Machine Learning Algorithms: ML algorithms were trained using large-scale datasets, encompassing diverse patient populations and clinical scenarios, to ensure robust predictions and evidence-based recommendations.
- Decision Support Tools: Softwarium developed user-friendly CDSS tools that incorporated contextually relevant healthcare research, aligning with clinical guidelines and supporting healthcare professionals in making medical decisions.
- Validation and Testing: Rigorous testing was conducted to ensure the accuracy and reliability of the CDSS. Clinical teams provided valuable feedback, enabling iterative improvements to enhance usability and performance.
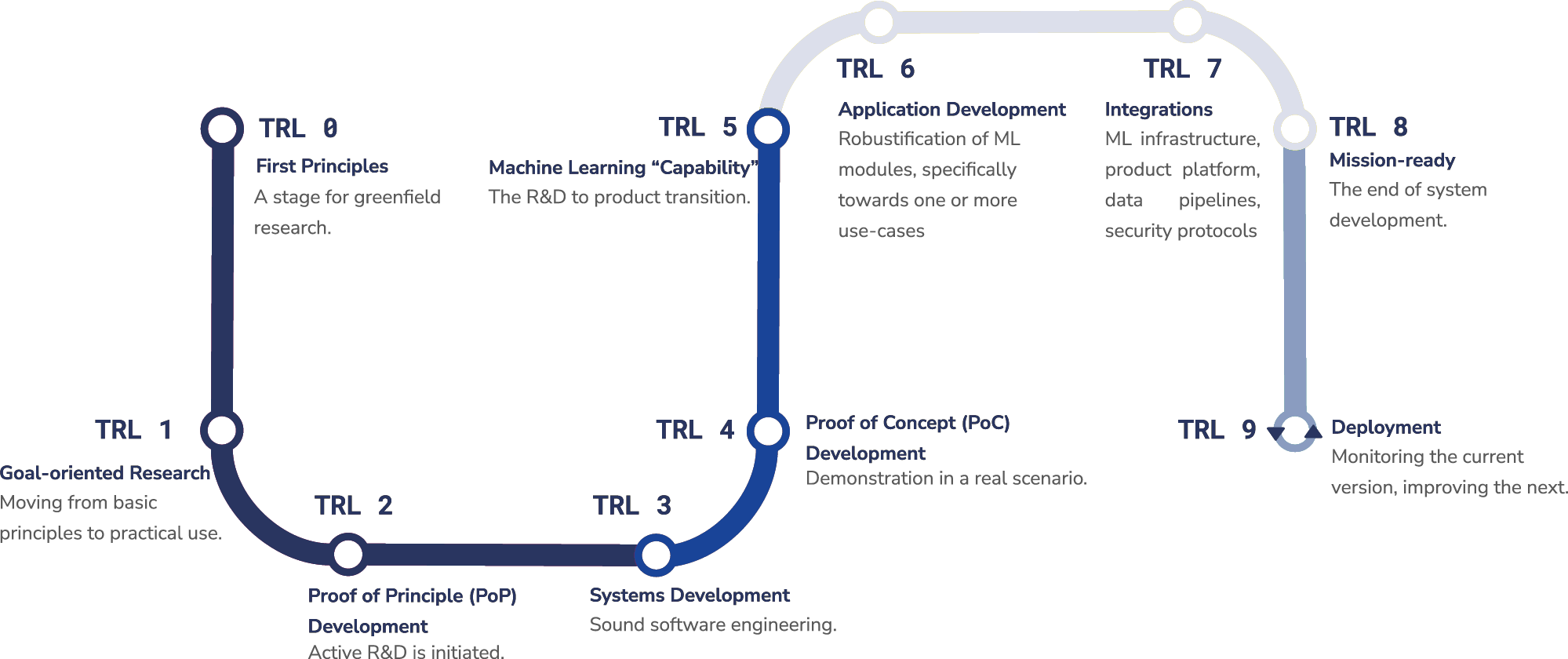
Results
The implementation of the CDSS yielded significant results:
- Improved Patient Safety: The CDSS effectively identified risk factors and drug-disease interactions, reducing the potential for medical errors.
- Enhanced Clinical Decision Making: Clinical team benefited from evidence-based recommendations derived from ML algorithms, enabling more accurate diagnoses and treatment plans.
- Streamlined Clinical Workflow: The integration of the CDSS with existing systems facilitated seamless access to patient-specific data and contextually relevant reference information. Improved efficiency and decision-making ultimately leads to reduced healthcare costs through increased productivity.
Client's Testimonial
"The CDSS developed by Softwarium has transformed our clinical practice: it enhanced the safety of patients and boosted our ability to make evidence-based decisions. It has greatly improved overall diagnostic accuracy and treatment outcomes. Softwarium's expertise in ML and their understanding of clinical workflows were invaluable during the implementation process."
Conclusion
By developing a powerful CDSS that seamlessly integrates with electronic health record systems, we have helped healthcare organizations improve safety, optimize making clinical decisions, and enhance patient aftermaths.
Our expertise in Machine Learning, AI, and health information technology innovations has facilitated the delivery of contextually relevant decision support tools that empower healthcare professionals to make informed clinical decisions based on the latest clinical regulations and patient-specific data.
Why Choose Softwarium?
At Softwarium, we are committed to revolutionizing healthcare through AI-driven solutions. If you are seeking to improve patient care, streamline clinical workflows, and enhance clinical decision-making, our team of experts is ready to collaborate with you.
Contact us today to explore our innovative CDSS and unlock the transformative potential of ML and AI in healthcare. Together, we can explore new AI prospects and a more efficient future for healthcare organizations and patients alike.
IMPLEMENTATION OF A CLINICAL DECISION SUPPORT SYSTEM (CDSS)
in Psychiatry Based on Machine Learning (ML) and Reliable Datasets